OpenAI’s sCM: Revolutionary Image Generation
Breakthrough in AI image generation technology offering unprecedented speed and efficiency
50x Speedup
OpenAI’s new sCM model accelerates image generation by 50 times compared to traditional diffusion models.
Fast Generation
High-quality images generated in just 0.11 seconds on a single A100 GPU without inference optimization.
Comparable Quality
Produces samples with quality comparable to leading diffusion models, using only two sampling steps.
Efficient Compute
Uses less than 10% of the effective sampling compute compared to traditional diffusion models.
Training Scalability
Scales to 1.5 billion parameters on ImageNet at 512×512 resolution, setting new training benchmarks.
Real-Time Potential
Opens possibilities for real-time generation across image, audio, and video domains.
OpenAI has unveiled a groundbreaking advancement in AI image generation that promises to revolutionize the field. Their new model, called simplified continuous-time consistency model (sCM), can generate high-quality images up to 50 times faster than previous methods, while maintaining comparable quality. This development could have far-reaching implications for various industries, from entertainment to healthcare, by making AI-generated imagery more accessible and practical for real-time applications.
The Breakthrough: sCM Model
The sCM model represents a significant leap forward in the field of AI image generation. Here are the key aspects of this innovation:
-
Unprecedented Speed: sCM can generate images in just two sampling steps, compared to the hundreds of steps required by traditional diffusion models. This results in a dramatic speedup of the image generation process.
-
Maintained Quality: Despite the substantial increase in speed, the quality of the generated images remains comparable to those produced by slower methods. sCM samples are within 10% of traditional diffusion models on standard quality metrics (FID scores).
-
Computational Efficiency: sCM uses less than 10% of the computing power required by traditional methods, making it significantly more efficient.
- Scalability: The researchers have successfully scaled the sCM model to 1.5 billion parameters, trained on ImageNet at 512×512 resolution.
How It Works
Unlike traditional diffusion models that gradually denoise images through many steps, sCM takes a more direct approach:
-
Direct Conversion: sCM aims to convert noise directly into noise-free samples in a single step.
-
Simplified Theory: The system builds on previous consistency model research but uses a simplified theoretical approach, enabling more stable training.
-
Distillation of Knowledge: sCM distills the knowledge of more complex models into a simpler, faster one.
Performance Metrics
To put the performance of sCM into perspective, let’s look at some key statistics:
-
Generation Time: The largest sCM model, with 1.5 billion parameters, can produce a single image in just 0.11 seconds on a single A100 GPU.
-
Quality Comparison: sCM samples are within 10% of traditional diffusion models on standard quality metrics (FID scores).
-
Scaling Properties: As both sCM and traditional diffusion models scale up, the relative difference in sample quality remains consistent, causing the absolute difference in sample quality to diminish at larger scales.
Potential Applications
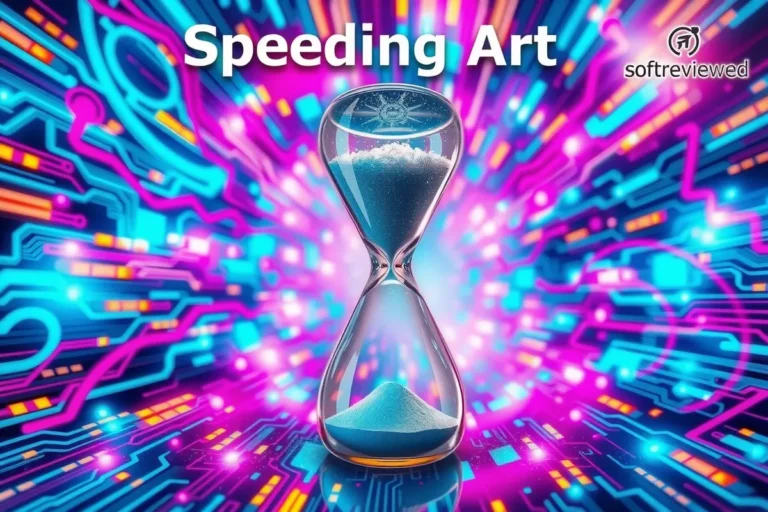
The dramatic speed increase offered by sCM opens up new possibilities across various industries:
-
Entertainment: Real-time generation of game environments, characters, and on-set visualization for film production.
-
Healthcare: Enhancing and generating detailed medical images for improved diagnostics and accelerating the development of new deep-learning tools in radiology.
-
Design and Creativity: Enabling rapid prototyping and iteration of ideas for designers, as well as creating interactive art installations.
- Real-time Applications: The speed of sCM makes it feasible for use in applications that require immediate image generation or modification.
Challenges and Limitations
While sCM represents a significant advancement, it’s not without its challenges:
-
Reliance on Pre-trained Models: The system currently relies on pre-trained diffusion models for initialization and distillation.
-
Quality Gap: There is still a small but consistent gap in sample quality compared to the teacher diffusion model.
-
Evaluation Metrics: The limitations of FID as a metric for sample quality mean that the actual quality of sCM-generated images may need to be assessed differently for specific applications.
Looking to the Future
The development of sCM is part of a broader trend in AI research towards more efficient and practical models. As we look to the future, we can anticipate:
-
Further Optimizations: Researchers will likely continue to refine these models, potentially achieving even faster generation times and improved quality.
-
Integration with Other Technologies: sCM could be combined with other AI advancements to create more sophisticated and versatile creative tools.
-
Democratization of AI Art: As these tools become faster and more accessible, we may see a surge in AI-assisted creativity across various fields.
Conclusion
OpenAI’s sCM model represents a significant milestone in AI image generation, offering a 50x speed increase without compromising on quality. This breakthrough has the potential to transform industries ranging from entertainment to healthcare, making AI-generated imagery more accessible and practical for real-time applications.
As we marvel at this technological achievement, it’s crucial to consider both the exciting possibilities and the ethical implications it brings. The future of AI image generation is bright, and it’s clear that we’re only scratching the surface of what’s possible with models like sCM.
Performance Comparison: sCM vs Traditional Diffusion Models
This chart compares key performance metrics between OpenAI’s new sCM model and traditional diffusion models, highlighting improvements in speed and efficiency.