📊 How Data Curation Boosts AI Performance
Explore the key roles of data curation in enhancing AI capabilities and reducing biases.
🔍Properly Curated Data
Properly curated data ensures high-quality, accurate outputs from AI models, enhancing their overall performance.
🎯Alignment of Data and AI Goals
Aligning data curation with AI model goals helps optimize AI performance and ensures better data selection for training.
⚖️Reducing AI Bias Via Curation
Data curation plays a crucial role in minimizing AI bias by identifying and removing biases in training data.
⏱️Enhanced AI Efficiency
Efficient data curation processes, such as automation and synthesis, reduce the time and effort required for AI model development.
📚Data Curation for Specialized Tasks
Data curation is particularly important for specific AI tasks, such as image recognition or natural language processing, where high-quality, annotated data is crucial for accurate outputs.
In the rapidly advancing world of artificial intelligence, staying ahead of the curve is crucial for businesses and developers alike. Enter DataChain, a groundbreaking open-source tool from Iterative that's set to transform the landscape of AI model evaluation and data curation. This innovative solution addresses a critical gap in the industry, offering a streamlined approach to handling unstructured data at scale. Let's explore how DataChain is poised to accelerate AI development and democratize advanced capabilities for engineers worldwide.
The AI Data Challenge: A Stumbling Block for Innovation
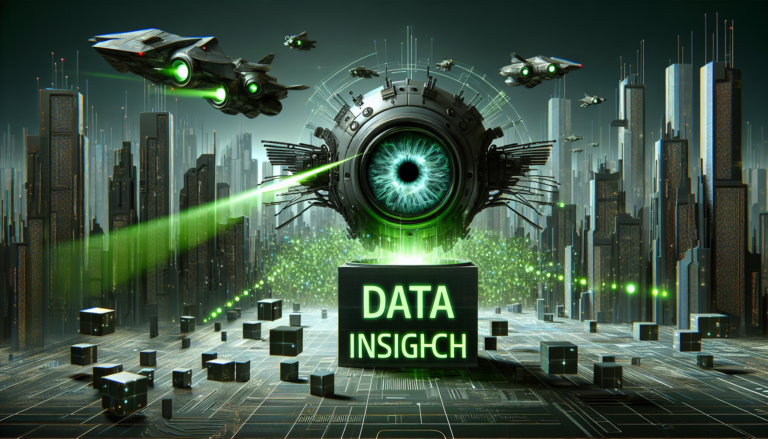
Before we dive into the specifics of DataChain, it's essential to understand the context of its creation. Despite the buzz surrounding generative AI, a surprising statistic reveals the current state of affairs:
Only 15% of surveyed companies have seen a meaningful impact from generative AI.
This low percentage is largely attributed to the difficulties in managing and processing large-scale unstructured data. As AI models become more sophisticated, the need for efficient data curation and evaluation methods has never been more pressing.
What Is DataChain?
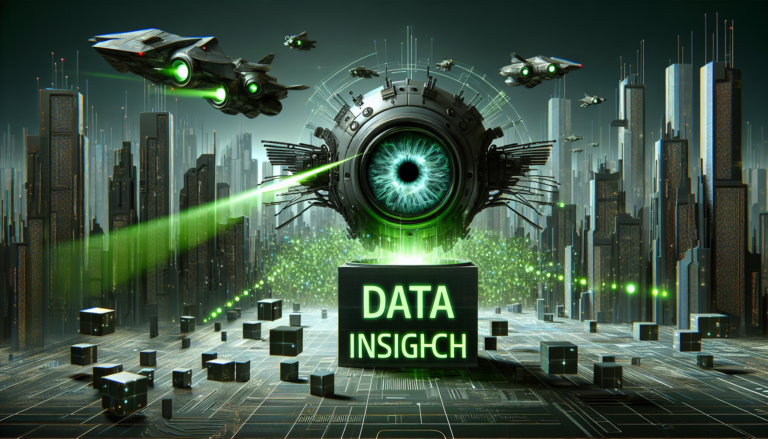
DataChain is an open-source tool developed by Iterative, designed to simplify and accelerate AI engineering workflows. Its primary functions include:
- Data curation at scale
- AI model evaluation
- Management of unstructured data
By providing these capabilities, DataChain aims to bridge the gap between traditional structured data technologies and modern AI workflows.
Key Features of DataChain
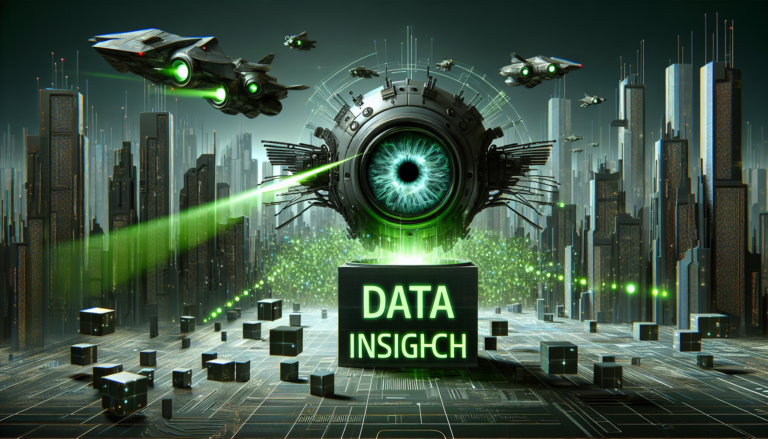
Advanced Evaluation Techniques
One of the most innovative aspects of DataChain is its ability to facilitate 'large language models (LLMs) judging LLMs.' This feature allows for more sophisticated and nuanced evaluations of AI models, pushing the boundaries of what's possible in model assessment.
Multimodal Generative AI Evaluations
DataChain supports evaluations across various modalities, making it a versatile tool for different types of AI applications. This flexibility is crucial in an era where AI is being applied to diverse fields, from natural language processing to computer vision.
Structured Data Support
The tool can efficiently store and manage Python object responses, utilizing the latest data model schemas from leading providers in the LLM and AI foundational model space. This feature ensures compatibility with cutting-edge AI technologies and facilitates seamless integration into existing workflows.
The Impact of DataChain on AI Development
Accelerating Workflows
By streamlining data curation and evaluation processes, DataChain significantly reduces the time and effort required in AI development cycles. This acceleration can lead to faster iterations and more rapid innovation in the field.
Democratizing Advanced Capabilities
DataChain levels the playing field for AI engineers by making advanced evaluation techniques more accessible. This democratization of technology has the potential to spur innovation across a broader range of organizations and individuals.
Enhancing Collaboration
The tool's ability to facilitate easier evaluation and comparison of different AI models promotes collaboration among engineers. This collaborative environment can lead to more robust and refined AI solutions.
Practical Applications of DataChain
Let's explore some real-world scenarios where DataChain can make a significant difference:
Natural Language Processing Projects: DataChain can help evaluate and refine chatbots, language translation models, and text summarization tools.
Computer Vision Applications: For image recognition or object detection models, DataChain provides a framework for assessing performance and curating relevant datasets.
Multimodal AI Systems: In projects that combine text, image, and possibly audio data, DataChain's versatility shines in managing and evaluating these complex systems.
- AI Model Comparison: Researchers and companies can use DataChain to benchmark different models against each other, making informed decisions about which models to deploy or further develop.
Overcoming Challenges in AI Development
DataChain addresses several key challenges that have historically hindered AI development:
Data Quality and Relevance
One of the most significant hurdles in AI development is ensuring the quality and relevance of training data. DataChain's curation capabilities help engineers filter and organize data more effectively, leading to better-trained models.
Scalability
As datasets grow larger and models become more complex, scalability becomes a critical concern. DataChain is designed to handle large-scale operations, allowing developers to work with massive datasets without sacrificing efficiency.
Evaluation Consistency
Consistent evaluation of AI models across different projects or teams can be challenging. DataChain provides a standardized framework for evaluation, promoting consistency and comparability in results.
The Future of AI with DataChain
As DataChain gains traction in the AI community, we can anticipate several exciting developments:
Increased AI Adoption
By simplifying the development and evaluation process, DataChain could accelerate the adoption of AI across various industries. This widespread adoption has the potential to drive innovation and efficiency in sectors ranging from healthcare to finance.
More Sophisticated AI Models
With better tools for evaluation and data curation, we can expect to see more sophisticated and accurate AI models emerge. These improved models could tackle increasingly complex problems and offer more nuanced insights.
Enhanced Collaboration in AI Research
DataChain's open-source nature and standardized approach to evaluation could foster greater collaboration in the AI research community. This collaborative environment may lead to breakthroughs that would be difficult to achieve in isolation.
Potential Challenges and Considerations
While DataChain offers numerous benefits, it's important to consider potential challenges:
Technical Complexity
Despite its aim to simplify AI workflows, DataChain and the associated AI foundational models may still require significant expertise. This complexity could potentially limit access for some engineers or organizations.
Regulatory Compliance
The use of AI models for data curation raises important questions about data ownership, control, and compliance with regulatory frameworks. Organizations will need to carefully navigate these issues as they implement DataChain.
Ethical Considerations
As AI becomes more deeply integrated into data curation and evaluation processes, ethical considerations around bias, fairness, and transparency become increasingly important. Users of DataChain will need to remain vigilant about these issues.
Expert Insights on DataChain
To gain a deeper understanding of DataChain's significance, let's consider this quote from Dmitry Petrov, CEO of Iterative:
"The biggest challenge in adopting artificial intelligence in the enterprise today is the lack of practices and tools for data curation and generative AI evaluations that ensure the quality of results."
This statement underscores the critical need that DataChain aims to address, highlighting its potential impact on the AI industry.
Implementing DataChain: Best Practices
For organizations considering the adoption of DataChain, here are some best practices to keep in mind:
Start Small: Begin with a pilot project to familiarize your team with DataChain's capabilities and workflow.
Invest in Training: Ensure your AI engineers are well-versed in DataChain's features and functionalities.
Establish Clear Evaluation Criteria: Define clear metrics and standards for evaluating AI models using DataChain.
Integrate with Existing Workflows: Look for ways to seamlessly incorporate DataChain into your current AI development processes.
Prioritize Data Quality: Use DataChain's curation features to maintain high-quality datasets for training and evaluation.
Collaborate and Share Insights: Engage with the broader DataChain community to share experiences and learn from others.
The Road Ahead: DataChain and the Future of AI
As we look to the future, DataChain represents a significant step forward in the field of AI development. By addressing critical challenges in data curation and model evaluation, it paves the way for more efficient, effective, and innovative AI solutions.
However, the true potential of DataChain will be realized through its widespread adoption and continuous improvement. As more organizations and developers incorporate this tool into their workflows, we can expect to see a ripple effect of innovation across the AI landscape.
The introduction of DataChain is not just about improving technical processes; it's about empowering AI engineers to push the boundaries of what's possible. As we continue to witness the rapid evolution of AI technologies, tools like DataChain will play a crucial role in shaping the future of artificial intelligence.
In conclusion, DataChain represents a powerful new tool in the AI developer's arsenal. By streamlining data curation and model evaluation, it addresses key challenges that have historically hindered AI adoption and innovation. As the AI community embraces and builds upon this technology, we stand on the brink of exciting new possibilities in the world of artificial intelligence.
Whether you're an AI engineer, a business leader, or simply an enthusiast of cutting-edge technology, keeping an eye on DataChain and its impact on the AI landscape will be crucial in the coming years. The journey of AI development is ongoing, and with tools like DataChain, that journey just got a lot more exciting.
Impact of AI on Data Curation and Development
This chart illustrates the significant effects of AI technologies such as DataChain and DELLM on data curation and development efficiencies.