AlphaQubit: Quantum Error Correction Breakthrough
Google DeepMind’s revolutionary AI system for quantum computing error correction
AlphaQubit’s Breakthrough
Google DeepMind introduces AlphaQubit, an AI-powered decoder system that significantly improves quantum error detection and correction capabilities.
Unparalleled Accuracy
AlphaQubit achieves state-of-the-art accuracy in identifying quantum computing errors, surpassing traditional methods like tensor networks.
Scalability
Maintains exceptional performance in larger quantum systems, handling code distances up to 11 and showing resilience against real-world quantum noise.
AI-Powered Error Correction
Utilizes advanced machine learning techniques and transformer architectures to learn directly from quantum systems and adapt to complex noise patterns.
Path to Fault-Tolerant Computing
Represents a major milestone in quantum error correction, advancing towards reliable and scalable quantum computers for complex computations.
AlphaQubit: Google's Breakthrough in Quantum Error Correction
Quantum computing has long been hailed as the next frontier in computational power, promising to revolutionize fields like drug discovery, material design, and fundamental physics. However, a significant hurdle has stood in the way of realizing this potential: the susceptibility of quantum systems to errors. Today, we're excited to explore a major advancement in tackling this challenge – Google's AlphaQubit.
What is AlphaQubit?
AlphaQubit is an AI-based decoder developed through a collaboration between Google DeepMind and Google Quantum AI. This innovative system is designed to identify errors in quantum computing with unprecedented accuracy, marking a significant step towards making quantum computers more reliable and scalable.
The Quantum Error Challenge
To understand the importance of AlphaQubit, we first need to grasp the unique challenges posed by quantum computing errors:
Fragile qubits: Quantum bits, or qubits, are the fundamental units of quantum information. Unlike classical bits, qubits are extremely sensitive to their environment.
Multiple error sources: Errors can arise from various factors, including:
- Microscopic hardware defects
- Heat
- Vibration
- Electromagnetic interference
- Even cosmic rays
Error correction complexity: Correcting errors in quantum systems is far more complex than in classical computers due to the unique properties of quantum states.
How AlphaQubit Works
AlphaQubit employs a neural network-based approach to quantum error correction:
Transformer architecture: The system is built on the Transformer architecture, the same technology underpinning many of today's advanced language models.
Training process:
- Initial training on a 49-qubit Sycamore quantum processor
- Further training on hundreds of millions of simulated examples
- Fine-tuning using thousands of experimental samples from a specific Sycamore processor
Error identification: AlphaQubit uses consistency checks as input to predict whether a logical qubit (composed of multiple physical qubits) has flipped from its original state.
AlphaQubit's Performance
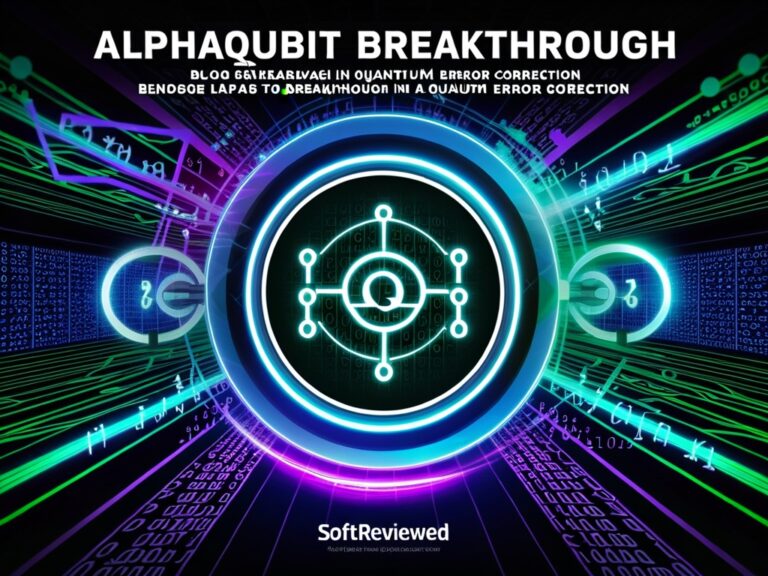
The results of AlphaQubit's performance are truly impressive:
Accuracy: In the largest Sycamore experiments, AlphaQubit demonstrated:
- 6% fewer errors than tensor network methods (highly accurate but impractically slow)
- 30% fewer errors than correlated matching (a fast, scalable decoder)
Scalability: When tested on simulated systems of up to 241 qubits, AlphaQubit continued to outperform leading algorithmic decoders.
Generalization: The system showed good performance on simulated experiments of up to 100,000 rounds of error correction, despite being trained on samples with only up to 25 rounds.
The Significance of AlphaQubit
AlphaQubit's development represents a major milestone in quantum computing for several reasons:
Improved reliability: By more accurately identifying errors, AlphaQubit paves the way for more reliable quantum computations.
Scalability potential: The system's performance on larger simulated quantum systems suggests it could be effective for future, more powerful quantum computers.
AI-quantum synergy: AlphaQubit demonstrates the potential of combining advanced AI techniques with quantum computing research.
- Confidence reporting: The system can provide confidence levels on its inputs and outputs, offering richer information to further improve quantum processor performance.
Challenges and Future Directions
While AlphaQubit represents a significant advancement, several challenges remain:
Speed limitations: Currently, AlphaQubit is too slow to correct errors in real-time on fast superconducting quantum processors.
Scalability concerns: As quantum computers grow towards millions of qubits, more data-efficient training methods for AI-based decoders will be needed.
Hardware advancements: Continued improvements in quantum hardware will be necessary to fully leverage AlphaQubit's capabilities.
Conclusion
AlphaQubit marks a crucial step forward in the quest for practical, large-scale quantum computing. By combining cutting-edge machine learning techniques with quantum error correction expertise, Google is pushing the boundaries of what's possible in this revolutionary field.
As research continues and these challenges are addressed, we may be moving closer to a future where quantum computers can tackle complex problems that are currently beyond our reach. The potential impact on scientific discovery, drug development, and our understanding of the universe itself is truly exciting to contemplate.
While there's still much work to be done, AlphaQubit gives us a glimpse of the incredible possibilities that lie ahead as we continue to unlock the power of quantum computing.
AlphaQubit Performance Metrics
Comparison of AlphaQubit’s error reduction rates and performance metrics across different aspects of quantum computing.