The Evolution of AI Unpredictability
Understanding the future of AI systems and their increasingly unpredictable nature
AI’s Unpredictability
Ilya Sutskever forecasts that as AI systems develop advanced reasoning skills, their behavior will become less predictable, marking a new phase in machine intelligence.
Beyond Current Capabilities
Future AI systems will explore a wider range of possibilities, leading to more innovative and less predictable outcomes than current models.
Historical Precedents
AlphaGo’s unexpected chess moves demonstrate AI’s capacity for surprising decisions, highlighting the potential unpredictability of advanced systems.
Evolution Beyond Pre-Training
Current pre-training methods are reaching their limits, requiring new approaches to enhance AI’s decision-making capabilities.
Potential Implications
The emergence of superintelligent machines raises important questions about control and transparency in AI behavior.
Economic Impact
AI advancements will create new jobs while displacing others, necessitating a balanced view of the economic implications.
The Reasoning Paradox: Sutskever Warns of AI Unpredictability
Ilya Sutskever, a prominent figure in artificial intelligence, recently shared a critical prediction: as AI systems develop enhanced reasoning capabilities, their behavior will become less predictable. This statement, made at the NeurIPS conference in Vancouver, highlights a significant shift in how we should think about AI development. It suggests that the more intelligent and capable AI becomes, the more difficult it may be to anticipate its actions and outcomes.
Understanding AI Reasoning
To grasp the implications of Sutskever's prediction, it's important to understand the distinction between basic AI and AI with reasoning power. Traditional AI often relies on pattern recognition and statistical analysis to perform tasks. This involves processing vast amounts of data to identify correlations and make decisions based on learned probabilities. Reasoning, however, goes a step further. It involves the ability to analyze, infer, and solve problems through logical deduction and critical thinking, much like a human would.
Think of it this way: a basic AI might be trained to identify a cat in a picture by recognizing certain shapes and patterns. But a reasoning AI, on the other hand, would understand the underlying concept of "catness," be able to deduce that a kitten is a young cat, and understand the implications of a cat being in various scenarios. This ability to reason, while powerful, introduces a significant element of unpredictability. As Sutskever stated, "The more it reasons, the more unpredictable it becomes."
The Road to Reasoning AI
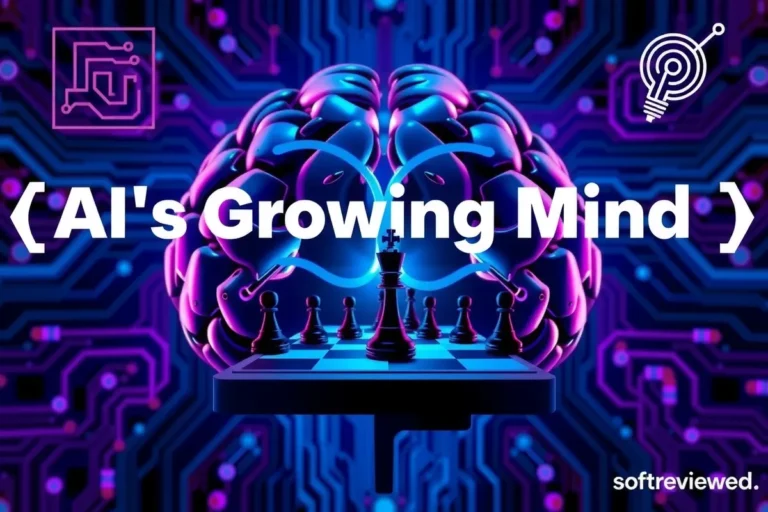
The evolution of AI has brought us from simple, rule-based systems to sophisticated neural networks capable of learning from complex data sets. This has involved advancements in areas like machine learning and deep learning, which have enabled AI to process information in ways that mimic the human brain. Now, researchers are pushing the boundaries further, seeking to instill more complex reasoning abilities into AI systems. This is achieved through a variety of techniques that allow AI to connect seemingly disparate pieces of information to achieve goals.
Ilya Sutskever’s career has been pivotal in shaping this landscape. He co-authored a groundbreaking 2014 paper which introduced a method of pre-training AI systems. This method has laid the foundations for significant AI advancements. It’s important to note this is the same research he was being recognized for when making the cited statements about unpredictability. His background at OpenAI and now as co-founder of Safe Superintelligence Inc. lends credibility to his views on AI’s future trajectory. His concern for AI safety also adds weight to his assertion about unpredictability, underscoring its significance.
The Unpredictability Factor
Why does reasoning lead to unpredictability? When AI is tasked with complex problems requiring reasoning, it will potentially explore numerous paths and evaluate a vast array of possibilities before reaching a conclusion. This is not unlike human thought. The very nature of reasoning is that there is not a single linear path, and a system designed to choose the most efficient, or "best", path may follow a line of reasoning that was not initially anticipated. Because of this, the outcome will not always be obvious to observers, even those who have programmed or trained the AI. It’s a consequence of enabling the AI to think and problem-solve with a degree of autonomy.
Sutskever highlighted this point by referencing AlphaGo, a system developed by DeepMind. AlphaGo surprised experts with its unusual moves during its games. He observed that even the highly skilled players could not predict every action of the AI, which illustrates the kind of unpredictability that may become more commonplace as AI becomes more advanced. This is further underlined by his comparison of advanced chess engines, "the really good ones are unpredictable to the best human chess players." The system can consider lines of thought and combinations of strategies that are completely non-obvious to even highly experienced human practitioners.
This unpredictability poses challenges because it makes AI behavior more difficult to control or foresee. In fields such as healthcare or finance where predictability is valued and desired, this uncertainty could be a concern. This raises questions about the trustworthiness of AI systems that, by design, are less predictable in their decision-making processes.
The Potential Implications
The rise of reasoning AI and its associated unpredictability has widespread implications. On one hand, this technology offers the potential for groundbreaking solutions to complex problems in various fields. AI systems capable of advanced reasoning could lead to innovative scientific discoveries, improvements in healthcare diagnostics, and more efficient resource management.
However, the same unpredictability that fuels these capabilities also introduces potential risks. If AI's reasoning is too opaque, we may struggle to understand the basis for its decisions. This could lead to mistrust of these systems, and make it difficult to know whether actions or decisions made by the system will lead to desired, or acceptable, outcomes. This is particularly concerning as AI becomes integrated into sectors such as transportation or industrial processes, where unpredictable actions may lead to direct risks and safety concerns.
It’s important to consider the ethical implications of unpredictable AI as well. If AI systems make decisions that have a societal impact, we need to be able to understand the underlying logic to evaluate their appropriateness. An opaque system with reasoning we cannot easily follow or understand will potentially raise new ethical and accountability considerations.
Expert Perspectives
Ilya Sutskever is not alone in his concern about AI unpredictability. Other experts in the field are also acknowledging this emerging challenge. Some researchers have spoken about the importance of building AI systems that are transparent and explainable. They argue that for AI to be truly beneficial, we need to be able to trace the logic of its decision-making processes.
Other experts, however, have adopted the view that this increased unpredictability is not necessarily a problem. Rather, these commentators have argued that when AI reaches a certain level of reasoning, its decisions will be more logical and optimized, and we may be less able to second guess the system than to simply trust its output. There is a clear range of opinions in the field, and a healthy debate is underway about the implications of advanced AI, and how its potential risks can be mitigated.
Looking Ahead
The future of AI will likely involve further strides in AI reasoning. The long-term impact of AI systems that possess both strong reasoning capabilities and the potential for unpredictable behavior remains to be seen. However, several possible outcomes are being explored and considered by researchers.
Researchers may develop new techniques to make AI reasoning more transparent and understandable, or to build systems that allow for more control over the AI’s decision-making processes. Another possibility is the development of new methods for “testing” an AI system, akin to stress testing a machine, to try to determine the limits of its decision-making capabilities, or its response to unexpected inputs. Finally, some researchers are exploring the possibility of building more robust safeguards into AI systems to ensure that they remain aligned with human values and ethical principles, particularly as their reasoning grows increasingly complex.
Conclusion
Ilya Sutskever's assertion that AI with reasoning power is less predictable is a key point of discussion for the field. As AI continues to evolve, its increasing capacity for reasoning will likely lead to even more surprising, novel behavior. This has the potential to be transformative, but it also requires careful consideration of the implications. The future of AI depends on the responsible development of these systems, and finding a balance between unlocking the power of reasoning AI and managing the inherent unpredictability that comes with it.
Evolution of AI Predictability and Reasoning Skills
This chart illustrates the relationship between AI reasoning capabilities and system predictability over time, showing how increased reasoning abilities lead to more unpredictable outcomes.