🔍 AI-Powered Financial Crime Detection
Advanced AI models are revolutionizing the detection of financial crimes and enhancing transaction security.
🤖 Advanced AI Models Enhance Detection
Large transaction models (LTMs) excel at finding novel criminal activities and reducing false positives, identifying authentic and illicit transactions alike by detecting patterns and anomalies.
📊 LTMs Over Traditional Models
LTMs offer higher resolution and natural language understanding, allowing them to detect complex relationships and patterns that traditional models often miss.
📉 Reducing False Positives
AI-driven false positive reduction models can achieve significant improvements, reducing false positives by up to 86%, ensuring more accurate threat detection.
🧠 Improved Contextual Analysis
AI applies dynamic rules and contextual filters to weed out false positive alerts, making it a more effective tool in anti-money laundering detection.
🛡️ Robust and Scalable Solutions
LTMs can be used as a foundational core infrastructure for new applications, evolving to address new challenges and use cases in financial crime prevention, including sanctions screening and circumvention detection.
The Rise of AI in Financial Crime Prevention
Artificial intelligence (AI) is making waves in the financial sector, particularly in the realm of crime detection and prevention. As financial institutions grapple with increasingly sophisticated criminal activities, AI models are emerging as powerful tools to enhance security measures and protect consumers. Let’s explore how these advanced technologies are reshaping the landscape of financial crime detection.
Understanding Large Transaction Models (LTMs)
At the forefront of this AI revolution are Large Transaction Models (LTMs), which are based on language models like GPT-4. These innovative systems treat financial transactions as if they were sentences, allowing them to identify patterns and anomalies with remarkable precision.
How LTMs Work
LTMs operate by:
- Analyzing transaction data as text
- Identifying complex patterns and relationships
- Detecting anomalies that may indicate fraudulent activity
- Reducing false positives in fraud detection
The ability of LTMs to understand and process transaction data in a way similar to natural language gives them a significant edge over traditional fraud detection methods.
The Impact of AI on False Positive Reduction
One of the most significant advantages of using AI models like LTMs in financial crime detection is the substantial reduction in false positives. False positives occur when legitimate transactions are flagged as potentially fraudulent, causing inconvenience to customers and unnecessary work for financial institutions.
Key Statistics
- LTMs have shown a 30% improvement in reducing false positives
- 70% of financial institutions now use AI and machine learning to combat fraudsters
These numbers highlight the growing importance and effectiveness of AI in the financial sector.
Advantages of LTMs Over Traditional Models
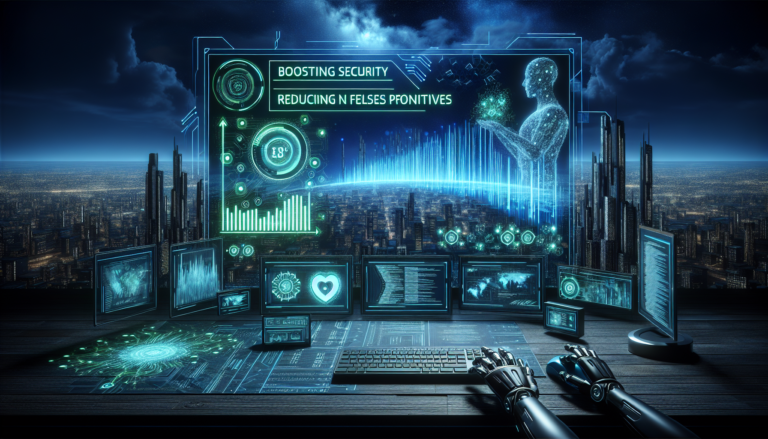
LTMs offer several benefits compared to conventional fraud detection systems:
- Higher resolution in data analysis
- Enhanced natural language understanding
- Ability to detect complex relationships and patterns
- Improved accuracy in identifying authentic transactions
These advantages make LTMs particularly effective in dealing with the nuanced and ever-changing nature of financial crime.
Improving Model Accuracy Through Continuous Validation
To ensure the reliability and effectiveness of LTMs, financial institutions employ rigorous validation processes:
- Tracking key performance indicators (KPIs) of LTM predictions
- Implementing automatic sampling for validation
- Continuously refining the model based on new data and insights
This ongoing validation process helps maintain the high accuracy of AI models in detecting financial crime.
The Role of Open-Source AI in Financial Security
The development and implementation of AI models for financial crime detection are part of a broader conversation about the role of AI in society. Mark Zuckerberg, for instance, has advocated for open-source AI to promote innovation and safety.
Benefits of Open-Source AI in Finance
- Wider access to advanced technologies
- Increased transparency in AI development
- Collaborative improvement of security measures
- Faster innovation in financial crime prevention techniques
By making AI technology more accessible, the financial sector can benefit from a collective effort to enhance security measures and stay ahead of criminal activities.
Challenges and Considerations
While AI models offer significant advantages in financial crime detection, they also come with certain challenges and considerations:
Data Quality and Model Collapse
AI models are highly dependent on the quality of data they are trained on. Recent research has shown that models trained on AI-generated data can quickly “collapse,” producing nonsensical outputs. This highlights the importance of using high-quality, diverse datasets in training financial crime detection models.
Balancing Innovation and Regulation
As AI technologies advance, there is an ongoing debate about how to balance innovation with necessary regulations. The financial sector must navigate this carefully to ensure that: new technologies are harnessed to improve efficiency and customer experience, while also safeguarding against risks such as fraud and data breaches. In this context, companies must stay agile, much like the financial sector’s rapid adjustments following ASML’s Q2 earnings surge, which reflected the broader tech industry’s growth. Balancing proactive regulation with innovation will be key to maintaining industry stability and fostering trust in AI-driven solutions.
- AI models are used ethically and responsibly
- Customer data is protected
- Regulatory compliance is maintained
- Innovation is not stifled by overly restrictive policies
Real-World Applications of AI in Financial Crime Detection
Let’s look at some practical applications of AI models in the fight against financial crime:
Transaction Monitoring
AI models can analyze vast amounts of transaction data in real-time, flagging suspicious activities for further investigation. This includes:
- Unusual spending patterns
- Transactions in high-risk jurisdictions
- Rapid succession of small transactions (smurfing)
Anti-Money Laundering (AML)
AI enhances AML efforts by:
- Identifying complex money laundering schemes
- Reducing manual review time for suspicious activity reports
- Improving the accuracy of customer risk assessments
Fraud Prevention
AI models can detect various types of fraud, including:
- Credit card fraud
- Identity theft
- Account takeover attempts
- Synthetic identity fraud
The Future of AI in Financial Crime Detection
As AI technology continues to evolve, we can expect to see even more advanced applications in financial crime detection:
Predictive Analytics
Future AI models may be able to predict potential financial crimes before they occur, allowing for proactive prevention measures.
Cross-Institutional Collaboration
AI could facilitate secure information sharing between financial institutions, creating a more robust network for detecting and preventing financial crime.
Integration with Blockchain Technology
Combining AI with blockchain could provide enhanced transparency and security in financial transactions, making it even harder for criminals to exploit the system.
Expert Insights
Let’s hear from some industry experts on the role of AI in financial crime detection:
“The LTM is a booster for what we’ve been doing over the years, enhancing our ability to reduce false positives and find more crime.” – Wolfgang Berner, CPO of Hawk
This quote underscores the transformative potential of AI in augmenting existing financial crime detection efforts.
Preparing for an AI-Driven Future in Finance
As AI continues to play an increasingly important role in financial crime detection, institutions and individuals alike need to prepare for this new landscape:
For Financial Institutions
- Invest in AI training for staff
- Collaborate with AI developers and researchers
- Develop clear policies for AI implementation and use
- Stay informed about regulatory developments related to AI in finance
For Consumers
- Understand how AI is used to protect your financial transactions
- Be aware of the latest financial fraud techniques
- Regularly monitor your accounts for any suspicious activity
- Use secure and up-to-date technology for financial transactions
Conclusion
AI models, particularly Large Transaction Models, are revolutionizing financial crime detection. By significantly reducing false positives, improving accuracy, and enhancing the ability to detect complex criminal patterns, these technologies are making financial systems more secure for everyone.
As we move forward, the continued development and responsible implementation of AI in finance will be crucial. While challenges remain, the potential benefits of AI in combating financial crime are immense. By staying informed and adapting to these technological advancements, we can all contribute to a safer and more secure financial future.
Remember, while AI is a powerful tool in the fight against financial crime, it’s not a silver bullet. Maintaining a combination of advanced technology, human expertise, and vigilant practices will be key to staying ahead in the ongoing battle against financial criminals.
Stay informed, stay vigilant, and embrace the positive changes that AI is bringing to financial security. Together, we can build a more secure financial ecosystem for everyone.
AI Usage in Financial Crime Detection and Prevention
This chart illustrates the adoption and perception of AI in financial crime detection across various aspects of the industry. Hover over each bar for more details.